When we imagine the help that artificial intelligence will give to the well-being of mankind, one of the most obvious and unquestionable topics concerns the possibility of discovering new drugs. In an increasingly digital and robotic world, it is in some ways suggestive that algorithms and artificial models are being used to solve problems that are typical of our biological nature.
In this context, DeepMind‘s research, with the release of the AlphaFold2 artificial intelligence system, goes right at the heart of the matter.
DeepMind is a company set up in London ten years ago to develop innovative AI systems, which quickly entered the radars of major investors. When Google acquired it in 2014, for $500 million, it did so by outbidding Facebook, which was also interested in the company created by Demis Hassabis, Shane Legg and Mustafa Suleyman. Two years later, in 2016, DeepMind made headlines for AlphaGo, the AI system that beat the undisputed Go champion – Lee Sedol – in a challenge watched by more than 200 million people worldwide.
Already during that challenge, Demis Hassabis, CEO of DeepMind, thought that the technology they had created could also be used to solve one of the thorniest problems in biology: predicting protein folding from the sequence of amino acids.
A long-standing problem
Knowing the structure of a protein means understanding what it does. A protein is made up of a series of amino acids that fold back on themselves in a complex manner and in numerous different ways. Imagine an enormously complex structure, where a fold in one direction rather than another implies a completely different behaviour of the protein. Seeing how the amino acids have folded means that we can see how the protein works, for example how it would behave if it came into contact with a given drug. In other words, protein folding is at the heart of biochemistry.
To make a real-case example, vaccines against Covid-19 are based precisely on the Spike protein which is present on the surface of the SARS-CoV-2 virus, giving it that ‘crown’ effect, the structure of which has been identified in recent months using traditional methods (although DeepMind has also tried to help out with its AI models). It is precisely by discovering the structure of the protein that vaccines were made possible.
Beside that, protein misfolding is thought to be at the root of various diseases such as Alzheimer’s and type 2 diabetes mellitus, Parkinson’s disease and cystic fibrosis. Understanding the structure of protein crystals could be the key to creating targeted treatments.
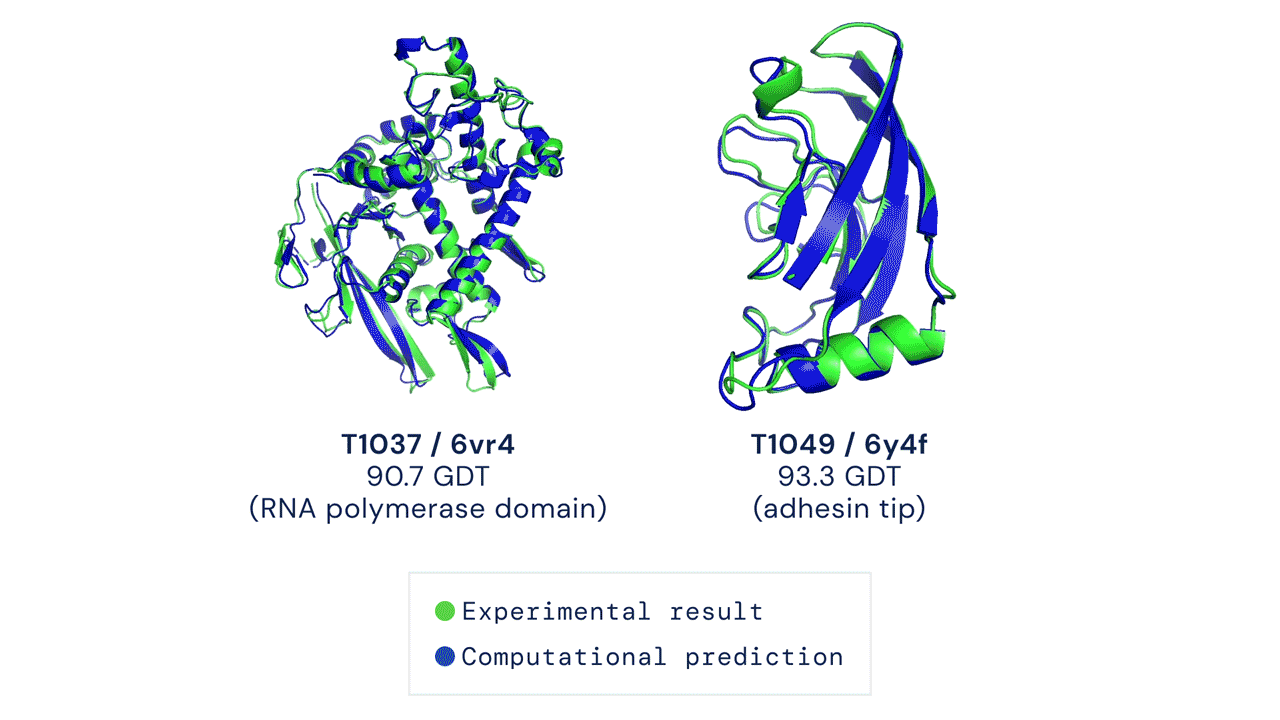
But what are the traditional methods used so far, and why was there a need for improvement?
Currently, three main technologies are used to study the structure of a protein: X-ray crystallography (used since the 1950s), cryoelectron microscopy and nuclear magnetic resonance spectroscopy. They are all valid techniques (the SARS-CoV-2 spike protein was found using cryoelectron microscopy) but have two major drawbacks: they are slow and very expensive. So slow and expensive that we are still left without information on the structure of most existing proteins. There are also a number of proteins, such as membrane proteins, which are difficult to crystallise and therefore to determine experimentally.
The CASP
For all these reasons, in 1994 the computational biologist John Moult and other colleagues created CASP, Critical Assessment of protein Structure Prediction, a series of experiments (but perhaps it would be more correct to call them competitions) in which various teams compete to identify, through ad-hoc software, the folding of a series of proteins whose structure is already known but not published. The software that comes closest to the real protein structures wins the competition. The aim of the organisers is obviously to stimulate research into this problem and to find software systems that can replace the methods used so far.
CASP has been held every two years since 1994 (with an additional special edition held this year to analyse SARS-CoV-2) and in 2018, at CASP 13, DeepMind presented its AlphaFold system winning the competition. Despite the win, however, AlphaFold did not even come close to the goal of 90 GDT_TS on average, which is considered the milestone beyond which the software’s performance is comparable to that of traditional methods. The first version of DeepMind’s software (which in the meantime has made its code public on GitHub) achieved an average GDT_TS of 68.5, enough to secure first place but a long way from the target of 90 that would make the model interesting for biologists and researchers studying protein folding.
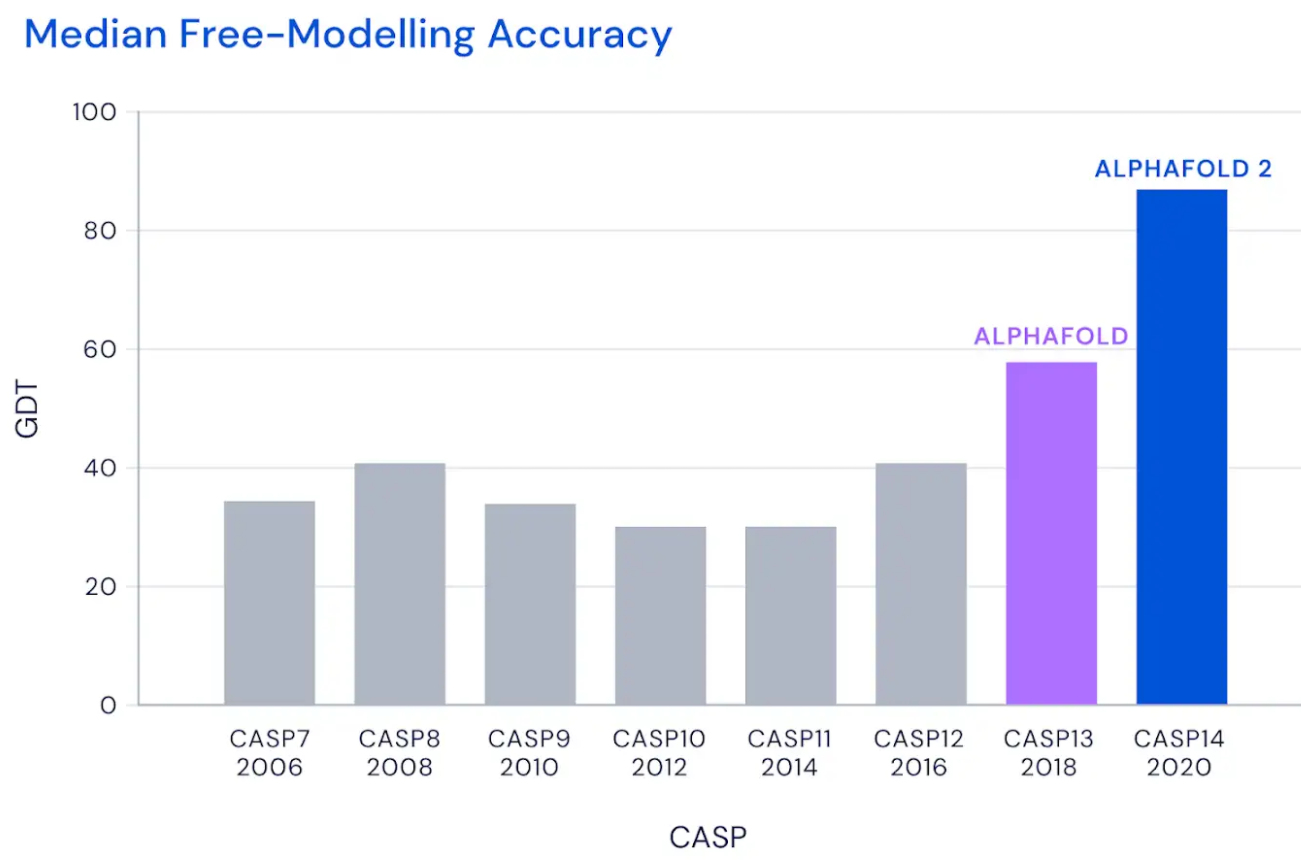
AlphaFold2
This year, however, at CASP 14, DeepMind unveiled a completely redesigned piece of software. AlphaFold in 2018 was essentially a set of fairly standard models (the main one was an image classifier), as project leader John Jumper explains in a Fortune article: one model predicted the distance between amino acid pairs, another model tried to determine the most likely angles, and finally the third improved the overall structure.
AlphaFold2, on the other hand, was designed from the ground up with the specific aim of predicting protein structure. The system no longer uses three separate neural networks: it takes the genetic information as input and outputs the protein’s 3D structure, achieving a median score of 92.4 GDT in CASP 14 tests, which surpasses Moult’s threshold of 90 and allows the software to establish itself as a real alternative to traditional methods. With the obvious advantage that predicting protein folding by AlphaFold2 requires only a few days (sometimes a few hours) and far fewer resources, compared with months or years of experimental analysis.
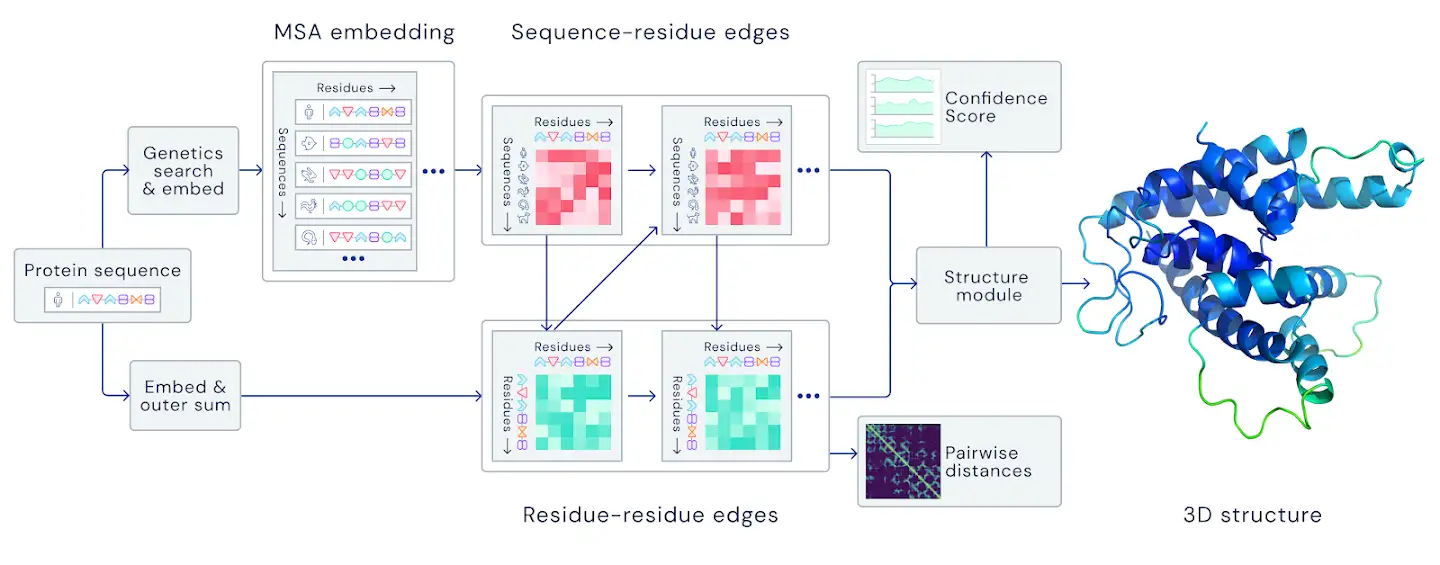
DeepMind trained the system on public data of about 170,000 protein structures, along with large databases containing protein sequences of unknown structure. AlphaFold2 used about 16 TPUv3s (i.e., 128 TPUv3 cores, roughly equivalent to about 100 or 200 GPUs) in the training, and the training time was a few weeks.
The reactions
There were many enthusiastic reactions in the research world, including Moult himself, who pointed out that after AlphaFold’s good result in 2018 this year at least half of the participating teams have started using some form of deep learning. Most of the teams came from academia, but tech companies such as Tencent and Microsoft have also decided to participate in CASP 14.
Structural biologist Venki Ramakrishnan, winner of the 2009 Nobel Prize in Chemistry, called the AI system ‘a stunning advance on the protein folding problem‘, which occurred decades earlier than many experts in the field had predicted.
According to dame Jane Thornton, former director of the European Institute of Bioinformatics at the European Molecular Biology Laboratory and an expert on protein structures, as quoted by MIT Technology Review, the level of efficiency achieved by DeepMind’s system could pave the way for mapping the entire human proteome, i.e. the set of proteins inside our bodies. Today, only a quarter of these are used as drug targets, leaving the remaining 75 per cent available for new discoveries, creating huge opportunities to study new medicines.
For many artificial intelligence experts and enthusiasts, the results achieved by AlphaFold are just one example of the vast opportunities we can explore with AI, trying to improve our lives, speed up scientific progress and deepen the understanding of biochemistry. And, as it happened billions of years ago, it all starts with proteins.